Exploring the limitations of AI in predicting the solar magnetic field
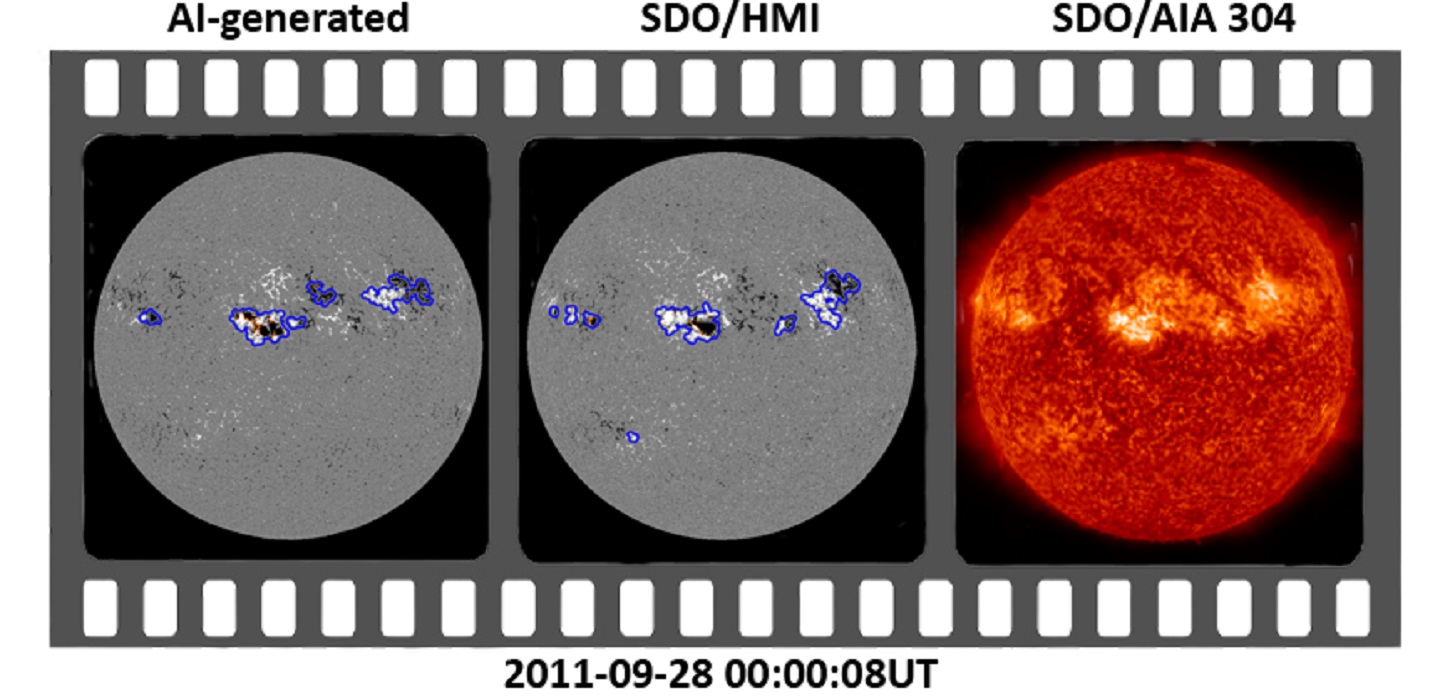
AI-generated, observed magnetograms and EUV images of the Sun. Photo credit: Liu et al. Nature Astronomy, 2021
13 February 2021
Scientists need to be aware of the limitations of Artificial Intelligence techniques used to predict the global solar magnetic field and space weather forecasting, according to a new academic study published today (Friday 12 February 2021) in the journal of Nature Astronomy.
Post-Doctoral Research Assistant, Dr Marianna Brigitta Korsos from the Solar Research group based in the Department of Physics at Aberystwyth University contributed to the collaborative study led by University of Sheffield and together with Queen’s University of Belfast, Chinese Academy of Sciences and University of Science and Technology of China.
In recent years, a number of novel AI techniques have been introduced to the area of solar physics and space weather forecasting.
For example, an advance AI model has been proposed to generate the photospheric magnetic fields observed by NASA’s SDO satellite mission using observations of the solar chromosphere with temperatures ~50,000 degrees as the only inputs.
Promising results have been achieved with a high level of cross-correlation between the total unsigned magnetic flux of the observed and the generated magnetic fields of the visible surface of the Sun, called the solar photosphere.
The photospheric magnetic fields of the Sun play a fundamental role in forming sunspots, for example, or the very dynamic regions called the active regions in the solar atmophere.
These regions are also believed to host the onset of coronal mass ejections (CMEs) and one of the largest and most energetic explosions in our Solar System, the flares. These gigantic eruptive features are the most dominant drivers of severe space weather events.
Space weather is a term that refers to the physical (e.g., magnetic and plasma) conditions in the space near the Earth, which determines the functioning and safety of a wide range of modern high-tech based facilities including airplanes, satellites, GPS, power grids and oil pipelines.
“However,” said Professor Robert Erdélyi, head of SP2RC, “mathematics and physics are the basics. It is always somewhat dangerous to ignore the mathematical modelling of the physics behind these solar activities when trying to predict them using modern techniques, such as AI. We must not use these fascinating tools as black boxes as this may mislead us and one may arrive at over-interpreted conclusions.”
By performing innovative and detailed analysis, applied mathematicians Drs Jiajia Liu (former Research Associate at University of Sheffield, currently at QUB) and Yimin Wang (University of Sheffield) as equal first authors, Xin Huang (Chinese Academy of Science), Marianna B. Korsos (Research Fellow at Aberystwyth University), Ye Jiang (University of Sheffield), Professors Yuming Wang (University of Science and Technology of China) and Robert Erdélyi (corresponding author, University of Sheffield), have addressed the critical question of what the limitations of AI models have in predicting the solar magnetic field.
“The research was initiated by the need to validate results obtained by using AI in space weather forecasting. This is an exciting area of application in this rapidly developing interdisciplinary area. We all have our own thoughts and occasional reservations about how deeply we should rely on AI. I am very proud to be part of this collaboration as a young female researcher at Aberystwyth University and have learnt a lot about this fascinating area of science” said Dr Korsos.
Dr Huw Morgan said that “The use of AI in astronomy, and solar physics in particular, is growing rapidly, and is particularly important in the context of predicting solar flares and space weather forecasting. The danger is in blindly relying on AI without a proper analysis. We also need insight into the underlying physical mechanisms leading to solar flares – AI can help give us this understanding, or at least point in the right direction. This collaboration is very exciting for us: it is a crucial step in benchmarking AI performance in this field, and offers so much promise for the future.”
Drs Liu and Wang added: “We have discovered that though the AI model performed well in predicting the total unsigned magnetic flux, its performance was not as expected in predicting other parameters including the net flux and the polarity reversion lines, which are vital in space weather predictions.
“Fundamental physics lies behind the results. The theory of magnetohydrodynamics tells us that imaging observations of the chromosphere and corona do not provide sufficient information about the photospheric magnetic field polarities.”
Professor Erdélyi further noted: “AI is an advanced and rapidly developing technique that has wide applications in science and technology. However, users must be aware of the limitations, especially when applying it to scientific research. Without considering the fundamental mathematics and physics associated, this technique is limited to achieve reliable results even if one is using the most advanced AI or machine Learning.”
- Link to the research paper: https://www.nature.com/articles/s41550-021-01310-6
- See also: Aberystwyth scientists help forecast space weather